Executive Summary
Meet Our Panelists
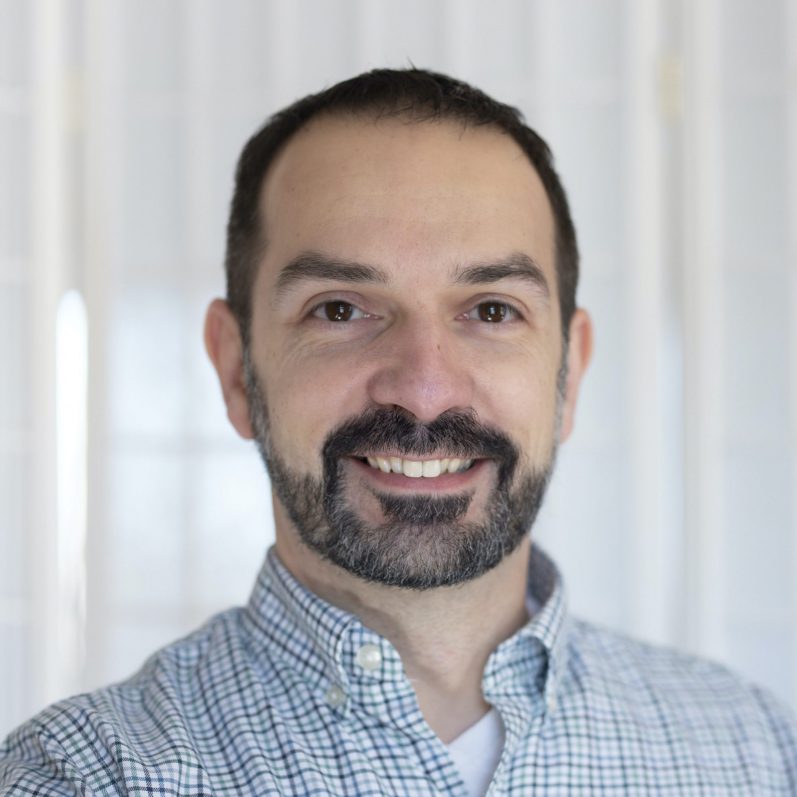
Dan DeAlmeida
Director of Product Management @ LabVoice
Dan DeAlmeida is the director of Product Management at LabVoice, where he works with a team developing a conversational voice assistant for scientists. Dan is a certified conversation designer and also has a chemistry background with over 20 years of experience working in a variety of laboratory environments implementing technological solutions from both the hardware and software perspective.
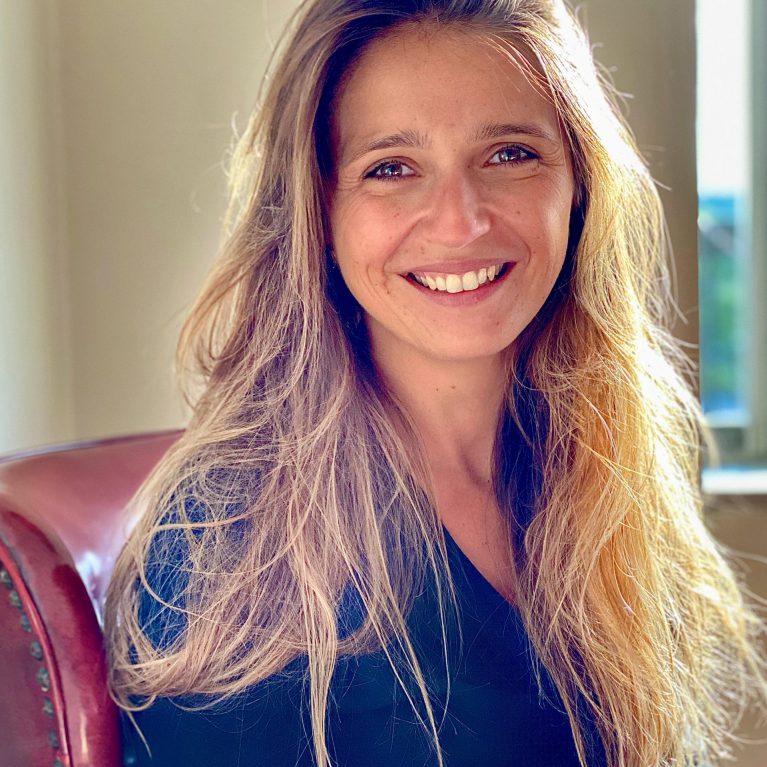
Alexia Sibony
VP, Product @ Orbita
Alexia has over 10 years of experience as a licensed physical therapist and in digital health product management. She has successfully piloted and scaled end-to-end product lifecycle for medical devices and SaaS solutions in compliance with U.S. and EU regulations. Alexia is currently the Vice President of Product at Boston-based digital health company Orbita, working to provide healthcare organizations with innovative conversational AI solutions that improve patient care experiences and outcomes. Alexia is also involved in Boston’s French-American community’s French-Tech Boston to help tech entrepreneurs.
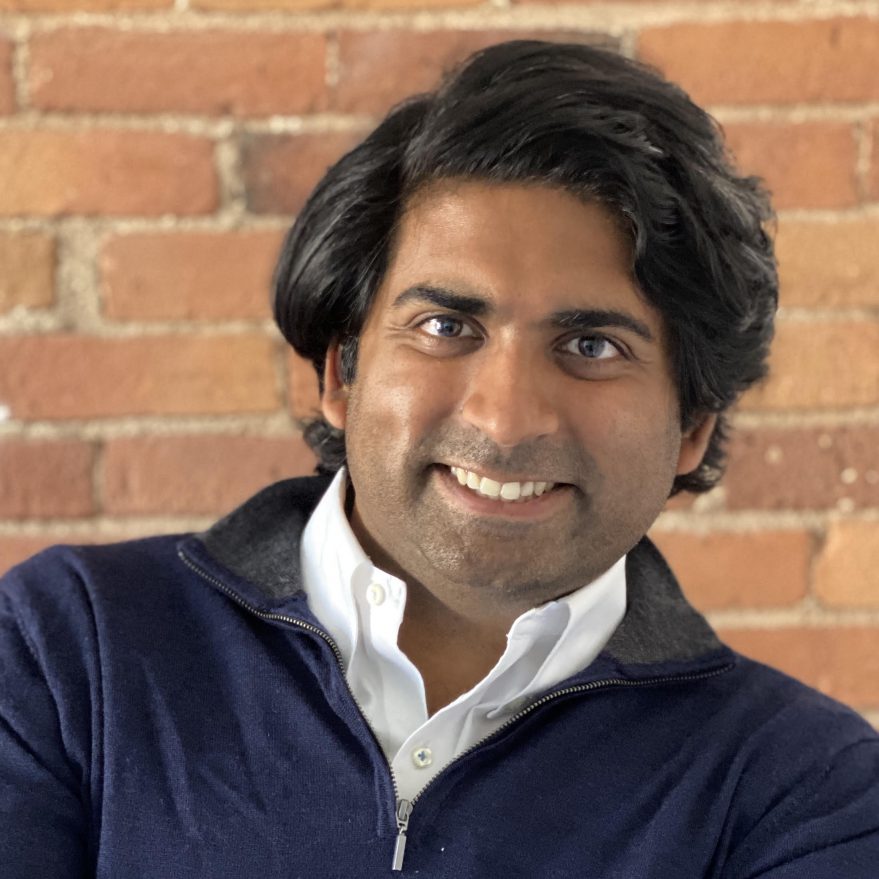
Rushi Ganmukhi
Founder & CEO @ Bola AI
Rushi Ganmukhi is the Founder and CEO of Bola AI, an AI-Voice assistant company in the healthcare industry. Bola AI's mission is to free-up healthcare providers from tedious documentation tasks and allow them to focus on patient care. Prior to Bola AI, he was a research assistant at the MIT Artificial intelligence lab, conducting research in the areas of machine learning and language processing.
Meet Our Moderator
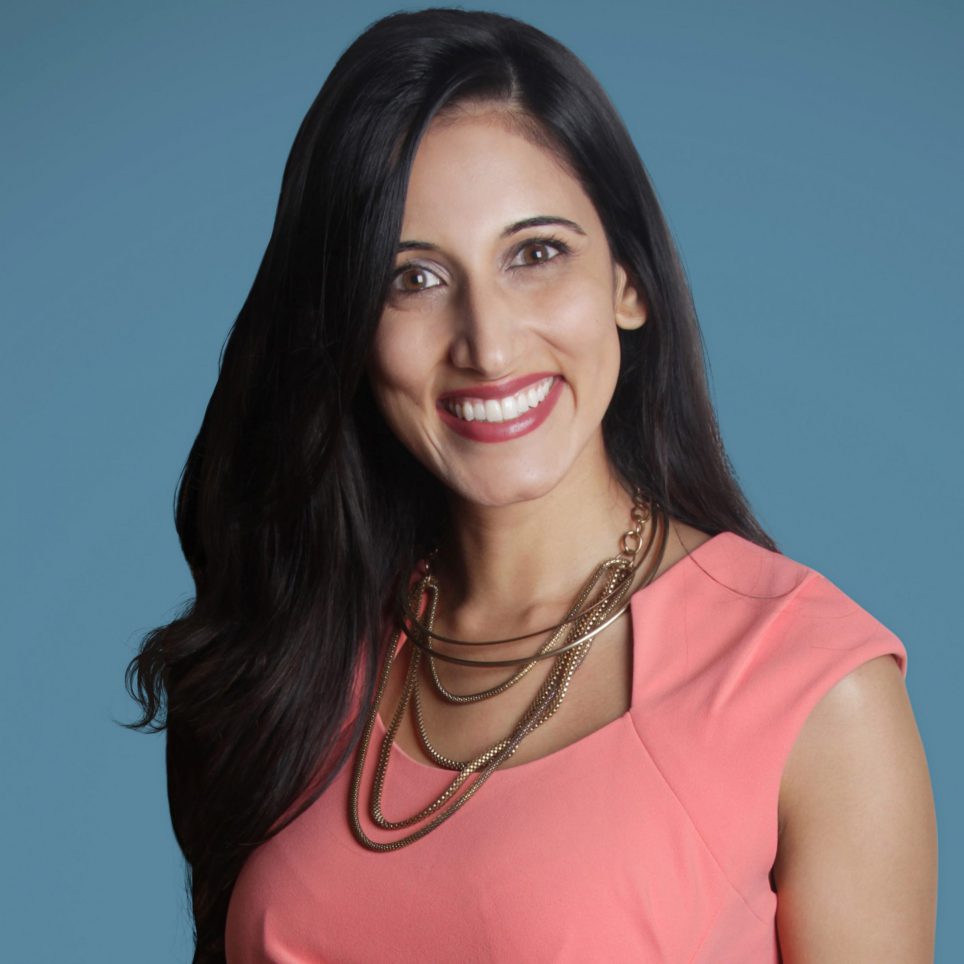
Sonia Talati
Senior Conversation Designer
Sonia has an M.A. in Journalism from Columbia University and a B.A. in Mass Communications and Economics from UCLA. She designed the conversation for the first HIPAA-complaint voice app at health-insurance giant, Anthem, and started her own company, HereAfter AI, developing, testing, and training "the world's first digital humans" as The Washington Post noted to help preserve people's life stories. She has written for The Wall Street Journal and Barron's and appeared on ABC-, NBC-, and FOX-affiliate news channels.
Watch The Event Again
Event Q&A
What are some of the aspects of conversational AI that you'd consider to be use cases in the healthcare space?
Dan: Our healthcare is more in the laboratory. So we're kind of preclinical, if you will, you know, helping scientists build you the vaccines, the drug products, or met devices. And our customers actually took us where we didn't expect to the biggest use case that we're seeing right now is onboarding and training. Because in the COVID area there, they need to be socially distant, so they can't have an experienced user next to a less experienced or a new hire. And they're using our voice assistant to actually walk them through their processes, which is great. I mean, something we didn't even think of, but it's very natural, you know, just as an experienced user needs to go through the process and have those documented next to them. In our use cases, the inexperienced ones can just get right up to speed very quickly by listening to someone tell them what the next step is, or asking them, you know, what the value is they need to collect.
Alexia: Conversational AI in healthcare, it's basically dillos for a more personalized and tailored experience, right so and it could be through multiple channels, whether that voice bot that can forward the experience over an analog phone or landline. We can also have chatbot experiences that can be initiated on your smartphone or through an SMS text or web browser from search, but more than ever, the most important thing with conversational AI in healthcare is basically the personalization. So we have the ability to adapt the entire user experience to a particular individual. And that's, to me the power of conversational AI more than any other technology that we've seen in the industry. And something that I like to remember is that the interface leverage language will learn at birth. And there's nothing additional interface to learn. Right? And that is, from a patient engagement, patient adoption a key concept.
Rushi: From our point of view, in terms of use case, we see, I guess, two distinct buckets. One is on the healthcare provider side. And that's where we work, I work mainly. And it's basically, in short, doctors spend way too much time entering data into their electronic health record software, they can spend around 50% of their time doing that. So voice assistants actually, for for a long time have been big in that space, I would say before this kind of 2016 really renaissance in that area. So that's the main provider facing use case. And then in terms of the consumer side, it's it can be things like apps, Alexa alerts to remind you of your medication. It could be pre-check-in. So I know a lot of dental offices we're working with, with COVID actually have implemented chatbot so that customers, patients sitting in their car can fill out forms, and they don't have to come and sit in the office that could be potentially dangerous.
Looking at conversational AI as a whole and then applying it to healthcare. How would you say that it's different for healthcare versus other areas or other industries that are beginning to implement it that was that we're seeing more and more these days?
Rushi: Yes, I'd say I think the biggest thing with health care is the importance of getting it right. It's okay if you ask for an Alexa command, and I get it incorrect. And it's what's the temperature outside? Right, that's, that's harmless, hopefully, harmless to everyone. But there's real importance on getting it right. And because of that, I think it's typically companies limit this limit the scope of what you can do. So you say we can handle these 10 things, but we can do them perfectly. And I think that's where a lot of places have started off. In terms of building for health, conversational in health care.
Dan: From my perspective, the challenge that we have is always a scientific term. And even the slang, right, you have to take into consideration the abbreviations that doctors or scientists use when they're speaking to each other, they've just created their own language if you will. And it's not the normal language that we're used to. So you have to take those things into consideration and make sure that your, your bot your AI, you know, whatever you have, as a system understands how to interpret that and react back to the user.
Alexia: If we think about why the specificities of the designing in healthcare, I mean, and you know that Sonia, right more than ever, HIPAA compliance, how do we design with HIPAA compliance in mind, and to maintain that high-security compliance, considering the patient health information, also called PH I, the tone of the experience as well, right? The way the interactions are presented to users is so very important. Actually, we were talking about that with Jennifer, the product designer at Orbita. And she said something very interesting. I like that, and I will quote her. Most people that use digital experiences to access healthcare are already in a stressed state, for one reason or another. So you have to craft the experiences that instill confidence and reassurance for them. So I think it's very important to consider as well when we are talking about patients and end-users to take their condition into consideration within the experience.
What are some of those specific challenges when designing for healthcare when you're actually designing those conversations? What are some of the biggest roadblocks you see are the biggest struggles?
Alexia: Something that is very important in our team at Orbita is to implement the user-centered design approach. So anything that we're doing includes the end-user, we're doing a lot of research with healthcare providers, patients, we really value that approach me as a physical therapist, more than ever, I want to bring the clinician and the patient into the process of designing. Because if you don't bring that in it, you miss the customer needs, you miss the interaction with the machine, and you really miss the context of the end-user. And, as we always know, always say, the value of conversational AI is that you can contextualize the experience, you can trigger educational content, you can trigger the right information to the patient, thanks to that context. And so it's important why we're designing conversational AI that we bring the users into the process of the development.
Rushi: Yeah, I agree exactly with what Alexia said, and the nice thing actually about bringing users in here is they don't need to speak your language, they don't need to speak product and what features you want, they literally, when they speak, they tell you what they want. So if you just listen. So for instance, yeah, we have an AI assistant for dental exams. So to start off with, we went to two weeks of dental exams, and we're listening to what they were saying we're writing scripts for what they were saying. And that was the exact product requirements that they needed. There was no translation needed between what they wanted and what they asked for. So it's, It was beautiful in that way.
Dan: So I agree with the user-centric approach, the extra challenge is knowing the environment, you know, you have to see them in their natural environment as they're trying the process. You know, so you do that happy path approach, you build it out, you give them a prototype to work with because there are things that they don't, they are not aware of that they're doing in their lab, you know, if their hands are dirty, or it's really noisy, that needs to be taken consideration. So those are some of the challenges are they handling, you know, in one case, animal health, you know, they're holding an animal, they can describe that to you. But until you see them holding an animal, juggling it in their hands while they're trying to talk, you might not fully experience that. So it's always super powerful, just work side by side with them, the user to make sure that it's working as expected.
What are some of the regulatory and ethical questions that you run into? Being in the healthcare space and dealing with conversational AI and new technology?
Rushi: I think it's HIPAA, HIPAA, HIPAA. It's a patient health information and what you do with it, where you store it, how secure you are, and it can be even for us to this day, our customers, it's, I would guarantee you it comes up in their top two questions when we first talked to them, are you HIPAA compliant? So it's a huge thing. And I think it's the number one law of the land, in healthcare. So be aware of that. And it's definitely a barrier to entry, but it doesn't necessarily need to be if you can be savvy about it. And I guess the second one that's not really pertaining to conversational is though if you get a little deeper into the AI, and a little more into the diagnostic space, you can fall under the thumb of the FDA regulation. So that's something to keep in mind as well.
Dan: I was gonna say we're entirely under FDA. So we're not in the HIPAA world yet. But the closer the more time goes by, you can see the lines kind of blurring. Because even the regulations that are from HIPAA, you can see them bleeding into the FDA in the lab world. And it's just from a personal perspective, right? It's making sure that everyone's information is private, even if the employees' information, all the data is secure, all the data is private and kept as is not accessible externally, or anonymized. So it's HIPAA, it's FDA, it's, you know, GDPR is a big push in Europe, to help secure all this privacy, private data of people. So I think we're all under that scrutiny in some way or another, our labs do a validation approach. So they'll look at the regular regulations, and they actually put us through the tests, as we're implementing it. So it's an interesting approach.
Alexia: I would just maybe add some, some thoughts here. And also, because I'm a quality engineer, so I've been into the quality system and regulatory approach since my master 10 years ago, and I try to bring that concept into the product team at Orbita. And I work very closely with security the head of security at Orbita, in order to actually educate the team on the concept that it's not a one-time change. It's a concept that we are we all need to understand it's a discipline to have, right. So when we say HIPAA when we say, PHI, when we say GDPR when we say FDA, we are in the most regulated market, and we need to understand that in order to do that to create the right experience, otherwise, it won't be adopted by the clinicians or the patients. So I think I would just share that thought here with the designers and the audience today is that spend some time to read about that. Try to find the best documentation to understand what does it really mean, to design for healthcare.
You mentioned something interesting at the beginning about those two questions that customers always ask. Can you give us the questions again, and potentially the answers you usually have?
Rushi: Sure. So the first one is do you integrate into our electronic health record? So that's, that's a huge one. We base Yeah, that's the biggest one. In healthcare, like the proper medical, recently, it's become a lot easier with Epic and apple orchard and a lot of things like that in dental. I'd say dental maybe five years behind. So that actually is still a valid question is do you integrate? And then yeah, the second one is typically around security. It's where do you store stuff? Are you HIPAA required? compliant? Are you were a voice company. So are you always listening to us? those types of things.
Alexia: And maybe if I may, there's something also very interesting the fact that we're partnering with Amazon with Google, right, we're talking about those voice speakers, it's also important to, to bring into the discussion about regulatory those partners. Because you could be HIPAA compliant, your platform could be HIPAA compliant, but the speakers, they have to support and Amazon made one of the biggest announcements right last year about a speaker that can support HIPAA compliance experiences. So that was a big lift, a bigger announcement, and a beautiful opening, to bring those speakers into the patient's home.
How are you tracking what you're doing in your progression, using conversational AI in healthcare, and what are some of those key metrics?
Alexia: So first of all healthcare industry, I always think about data. It's a data-driven market. So you could not create an experience without having metrics in mind, and really define those metrics at the very beginning. And more than ever, you could have key indicators, I can give you and I will give you some examples. But it's very important that there are not always the same for every company. So it's very important to identify the client's business, the goals, the need of the users to adapt those metrics and create the right dashboards and Analytics reports. You have quantitative KPIs key performance indicators, and qualitative. I could give us 15 examples of quantitative but one of them would be a chatbot activity volume, For example, offering a conversational experience, increase user engagement. And the metrics would be total conversational experience visitors, returning visitors, new visitors, total sessions, that's one of the examples I had, you have the bounce rate as well. And from the qualitative KPI, then you have those comprehension levels, the Self Service rate. For example, when we were talking about earlier in the breaking room, the call deflection right and call it automation use case. That's, that's a great KPI. If you can reduce the first call resolution rate, that's one of the metrics we can see on that use case. And also you have the user feedback. And I know that one of the questions we will address afterward, but the user feedback is one of the most important metrics.
How are you collecting user feedback?
Rushi: I think the nice thing about conversational is you can both have implicit and explicit feedback. So the explicit feedback would be asking for it. So the nice thing again, with conversational is, it's faster than typing or anything like that. So taking a voice. Note from somebody rating, our product is very quick to do and very easy for them to do as well. And then in terms of kind of the implicit, it's, it's looking at the data under the hood, we have a lot of logs and other such things from usage. And we can see when the user is going wrong when they're going right, and even new things that they're trying. And the new things that they're trying typically, or they're trying to do but doesn't work on our system currently, that typically leads to new features in our product.
Dan: I mean, today to collect the user feedback, it's a little bit harder for me, I'm a very visual person that likes to watch, you know, and be there with my users. And we're not allowed in the labs anymore. Suddenly, essential personnel. So we've been doing a lot more video calls or having them actually just record the video while they're doing it. And it's super important because you just miss these little things. Like, I watched a user the other day speaking to his phone like this, not really not realizing that he had a headset on. And he was just talking to his phone because that's the natural way he talks to people on a phone. And it's just little simple things like that, that you can improve the quality of your system by trying to just take all those aspects. And again, it's all that environmental factors that go in there. So but today, we're doing a lot of video calls, pre-recording, or, or getting them to just record it for us. Any and any, you know, obvious any information that they can give us from a voice or just emails also how.
Because this is such a new technology, and there's often a learning curve for people just to get accustomed to having a conversation with a virtual assistant. And how much do you find the feedback? Is it valid?
Rushi: Yeah, in terms of user feedback, it's pretty funny. Yeah, we're a voice assistant. So what they do when our users have an issue is they say the microphones not working. And literally, that is everything that could be the entire stack is the microphones not working anything could one thing could be wrong. And it displays that to the user. So the good thing is they tell us, but then it becomes our job to really look into the data and understand where it's going wrong. But it can be, it can be difficult for a user to understand actually, what part of your system is breaking or having issues.
Alexia: Something that actually reminds me is that you could have the user feedback directly from the user. But in the future, the user feedback will come from the devices. And that will be from the motion sensors, from the different devices that the patient will wear, right? You could have sources of user feedback that are so various and multiple that once aggregated, you get a pretty strong report that can help improve the experience.
Dan: To me, I mean, you can consider what is valid or not valid feedback. And everything's valid if it's coming from the user to me, and listening to it. But you have to take that with a grain of salt because we are doing a voice assistant. And one of our modes is actually in the mobile app. So as you're speaking, you can see what you've said, you can see that what the assistant said and what you've said, Now take that into consideration that As humans, we translate how each other speaking, and taking consideration accents and slang and etc. I'm from the northeast. So occasionally I dropped my Rs. So the sense the question, What are my experiments? When I say it naturally is one of my experiments. You will see on the screen, what my experiments, it still knew what I wanted to do. Is it wrong? No, it's actually what I said how I said it. So you have to take those considerations and get them to trust the voice intention more than just looking at a visual because we don't see the visual of what everyone else is saying here.
What can users expect to be the biggest change for them when interacting with the healthcare space? Because of conversational AI, How is the world going to be different?
Dan: To me, I think, if we start looking at, I don't know, environmental factors, again, I keep saying that, but you start taking your conversational AI and you integrate it with all your sensors, you know, light or heat or humidity. Or even, you know, you take it a step further and start adding in some AR stuff in there. Now you have all this extra data that is powering your conversation with the user, right and now you can have a more natural experience. That's what we do as people. We're looking around, we're hearing things we're smelling. We're feeling different temperatures. And if we can augment the conversational AI with all this information, it's going to feel more natural and it's going to feel like you have a person there with you.
Alexia: It's a very good point, something that we do see already in the context recommendation in Jain. So based on those different factors, right, and the environment, the platforms are capable to support recommendations and contextualize the experience based on the environment, meaning, for example, you would not ask a question about your temperature. If the patient is sharing a symptom, the symptom checker would be triggered. And then based on the answer, you would get a specific conversational experience and you would even trigger a call to a call center emergency room or whatever we even see sentiment analysis when they write based on those thanks to the conversational AI models. So that's one of the future that envisions that there are so many.
Rushi: I think it's less efficient. And hopefully, for the end patient, it's less time. It's less time in the doctor's it's less time waiting in the waiting rooms. It's less time for them in those situations.
What are your thoughts on personality, and using that in building these conversational experiences?
Dan: It's huge, you don't want to work with someone who has a terrible personality, right? Yeah, it's nobody who wants to do that. So it's a super important factor, we don't have a brand personality, per se, we actually tailor the personality to whatever the end customer wants, it's really interesting, and they can have fun with it. They can make it as demanding as they want. We've had some people ask if they can curse, you know, it's up to them if they want to, you know, treat their employees that way or do in a joking way. It's, it's how you want the people doing the job interacting with your conversational AI feeling. You know, and that's really a huge factor there.
Alexia: There's something that we do see as well, I've been feeling that as a practitioner, actually, the empathy, We've always, as a condition, I've been trying to bring empathy with my patients. And one thing that we could actually bring into a conversational AI experience is empathy. We can a voice, the Sorry, sorry to hear that. Obviously, if you say, sorry to hear that. Obviously, it doesn't sound great, right? So the empathy in the conversation is key. And I'm coming back to the context as our as well, the sentiment analysis, the fact that we can actually bring that voice adapted to the context. And we, you would then say, the words with a more human-like, actually, Google just improve their, their quality of voice. And deep learning helps make the sound very in way more human-like today. So we are getting there into a world where a conversational AI, design, and experiences that will get more human, human-like, but it still has to, to feel like a bot, if it's a chatbot it, we should always be able to differentiate between the bot and the human. Otherwise, it could be problematic on many levels.
Rushi: Actually, Alexia, that's a great point. And that's something we really want to drive home with our users to is that this is a bot. It's not a human, it's a bot. So meaning it has limitations, and you need to learn those limitations and you need to play within that field. And that's actually something we didn't do really early on, we were just like, go use it as a voice assistant go wild. And then we quickly realize that that's not the case. That's not where the technology is yet. We have to limit our users right now.
Have you found any struggles with merging and dividing these personalities within the same conversation?
Alexia: We are actually doing that at Orbita, we're trying to segment the user, the user person as right, and do doing some A B testing. But the good thing is that we've taken into consideration the user-centered design approach. But then we can look into behavioral economics, we can look into behavior change psychology to really inform how different people relate to and adopt digital technologies differently. And so while we are working on those that user segmentation we learn a lot and we are capable to create specific conversational AI experiences.
Dan: We actually separate our users, we do it by their teams. So if you're working in one particular, you know, group, you're doing chemistry or biology or math devices, then each of those teams can develop their own personality, their own a system, to respond to how their team works, we do use a few user metrics in order to help you know, because at the beginning when you're a new user, you're probably going to need a lot more help from more verbose. And so you have that more of a trainer personality, as you become more experienced, you need less help, and because more of an assistant personality, so you kind of dropped through the chattiness of it. But the overall experience is segregated by sections.
What are some of the milestones you're looking forward to? Things you're excited about worried about? We would love to hear about that.
Rushi: So yeah, we think right now we really feel the conversational or voice in healthcare is it's really at the beginning. It's the early adopters who are using it, that probably everyone in the market is aware of it patients are aware of it healthcare providers, lab engineers, everyone's aware of it. But it's really only those early power users who are using it and going, going for it. So I think the biggest milestone is now really cracking into that middle portion of the market, your average users. And a lot of that work, actually, at least for us, what we're really focused on right now is the customer experience is how to make this product very easy to use. I mean, for a voice product, a simple thing, like how do you get help? How do you deal with something like that's a difficult problem to solve. So building in those things to really make this available to the middle of the market, I think is a big thing. That's what's on the horizon right now.
Dan: I agree. It's sorry. It is a very early adopter right now. I'll be excited when it is more in the middle. And people start-stop thinking of their voice assistant, or conversational AI as atomic questions. What time is it? What's on my agenda? What's the weather like? And they start thinking about, oh, I would I want to have this conversation as part of my day for when I'm making a cake, or when I'm doing a recipe or when I'm building a drug or working on my car, whatever it is. And once they start thinking of those use cases, naturally. It'll be super exciting for us.
Alexia: Yes, actually, I've been sharing the thoughts with the CEO of the company at RB that we've been discussing the future and he was actually talking about the 5g and obviously, the 5g will be a game-changer. I didn't realize how, how big that that connectivity and the network will ease right the experiences. So conversational AI will be everywhere. You'll have voice assistance, everywhere the devices will talk to you. And basically, the patient will become the hospital, the environment will be able to support its chronic disease, It's conditions. So that is one of the key milestones that We envision were setting up a voice speaker or setting up a device will be easier than easier than ever. And so that's that, and also very cheap, right and really less expensive than it is today. Also, we, we've seen more accuracy, and the fact that it's more accurate, the different The, the tools and the software's are capable to gather information to, to, to get more into the details of the patients bring an accuracy on top of 99%. We've seen that with one of our tools lately, the adverse event detection in the life sciences market, where you could have a 99% accuracy when it comes to detecting adverse events from a patient intent. So, yes, and then the third one was the fact that the thing that I mentioned earlier, right, the recommendation in giant, the fact that you would have more context-based experiences that will become natural, without even thinking about that. And it's key, because today, we could see that limitation within experiences where actually if you do not update the different educational content and protocols based on the different patients intent, how do you get the adoption required for long term treatment, we've seen that for digital therapeutics, we've actually heard that from a lot of companies now that digital therapeutics will start bringing conversational AI in their solutions to actually support the context-based experiences.
How challenging are the medical terms for ASR (Automated Speech Recognition) when you're building for consumer platforms, Google Amazon, and such?
Rushi: We build for health care providers. But the medical terms are difficult. I think one we see a lot. And just for instance, in dental is the word, Musial. And it comes in as museum all the time, and it drives us nuts or it used to. So the medical terms are definitely a hard problem to crack.
Alexia: It is a hard problem. We've actually tried to trigger the targeted problem at Orbita with an enzyme that we've we've built that can support multiple synonyms. And so once you create your knowledge base, and you make sure that it's supporting any, any type of uteruses, then you, you could work around that we'd say that difficulty with medical terms.
Dan: So we're very similar we so we build everything by the process. And so that process, therefore, has a context. And then when you get to a prompt, that prompt also has a tighter context. And then we are collecting data, that data is of a certain type and then you can load it with synonyms or vocabularies that you might be more aware of and your accuracy level goes up based on that but you still have the goofy you know sayings every once in a while you'll miss one or two and it sounds strange.
How do you account for a cultural bias globally, so different sets in different locations?
Dan: For us, we're starting to branch out a little bit more. We're actually trying to work in different languages we've tried out German and Italian and French we have a pretty diverse team. We've tried out Portuguese to just slightly more Brazilian Portuguese than anything else but we also try to have people speak in English and then set their locale to English with an Indian accent UK accent you know French accent and it actually improves the recognition a little bit more.
Rushi: Oh, no, yeah, we haven't done any work. Really with multiple accents outside the US.
Alexia: We had some, some great partnership and collaboration with us. With some chatbot experiences actually in Spanish and I'm trying to bring the French accent as well. But obviously, there's a from a back end perspective and how to support that it's a question of knowledge base and the fact that it's artificial intelligence, right, then it can learn, we can actually leverage learnings from one language to another and make sure that the conversational experience gets improved, thanks to those multiple languages. So it's pretty interesting actually.
With AI data is important. So does that put small startups at a disadvantage as compared to Facebook, Amazon, Google who possess terabytes? Who processed the terabytes of user data?
Rushi: For us, it was all about, I guess, quality of data and the specificity of data is in the field, we have no those companies actually don't have data in that field. And to get started, you basically have to bootstrap and you have to hustle. And you have to find data sources that are close, good enough that you can start to use to build a model, and then over time, really collect that data and grow from there.
Dan: But the big data warehouses don't do anything for us, because our industry is so specific. But a lot of our customers have data lakes they've been collecting for years if we can't leverage that because there's really not a lot of voice information there. As far as synonyms or pronunciations. We just have to build on top of what they already have.
So in terms of chosen solutions, do you prefer homegrown AI models, or using existing cloud providers to scale PLCs to the actual market?
Rushi: We're really homegrown stuff. We just for the exact market, we are we're in the exact context, we needed the exact vocabulary we needed, all homegrown.
Alexia: It homegrown as well.
Dan: It's the same.
Conversational AI means that there is a conversation. So how do you ensure that we don't over-communicate and get that right balance?
Sonia: I really try to imagine what these characters like, you know, what do they look like? If I were to meet them on the street? You know, what would they talk about? Where would they be heading? Just getting those colors as much as possible. It's almost like creating you know, it's like, a creating a virtual actor, it's like, Who is this person? And who do we need this person to be for this use case I'm dealing with patients might be very different than dealing with customer support. And just having that person in mind, I feel like helps, really, and craft messages that are in line with it. And so much of this requires intuition. Intuition plays a huge role, I think, and being able to feel out what makes sense.
Alexia: For sure, but also, when we see that in the life sciences market, right, with our pharmaceutical companies, they actually provide the content, they have a very, very strong process of content approval. And so it's, it's almost easier right to know the amount of content that needs to be to be provided to the user. But the difficulty then is to make it conversational and easy to read. And I would say user friendly. So that's why the user research and the user interviews, and afterward after prototyping is very important as well.
So how do you deal with feature discovery?
Rushi: One thing we do as well, the great thing about voices, you can here you can see what they've tried to do. But that didn't work. And if we see enough of those, if there enough of the time, they're trying to do one thing, but we don't support it. We know that's a feature. And we know, that's something we have to build.
Dan: I see that as how are you discovering, like, the invocations and how you're questioning it. We're trying some different ways, you know, when we do the onboarding, either take them to a tutorial or send them a cheat sheet beginning or just the word help, say, help as many times as you want. And then it triggers, you know, some more information about what you're looking to do. It's, it's, it's learning in-process mode, I guess, right now for everyone to understand, you know, not to have that visual interface and just ask for help.
Alexia: At Orbita, as we are a platform. Our feature discovery are also from a modules perspective and features of the platform. And we get actually a lot of feedback from our customers that are getting feedback from their end-users. So it's a process of gathering that user feedback, the end-user feedback, making sure that we can consolidate it from a platform perspective to deliver a feature that will support multiple conversational experiences, across multiple use cases. So it's, it's more from a SaaS platform perspective that we are building our process of discovering features.
Sonia: I would just add to that, one key area that I found to look for features is just what people are calling in for, if you're, if your client has a phone line that's receiving a high number of, you know, a high number of calls, what what are most of them, you know, and sometimes the numbers can be pretty surprising. They can be sometimes as high as 70%, for one particular type of call, you know, that's a feature that would be worth exploring for, you know, virtual assistant. And so just being able to sort of replicate that call experience through virtual assistant is an area that I've found to have a plentiful number of features to explore.
Curated Resources By Voice Tech Global
NLP For Healthcare
In this article, the author shares some key takeaways of building natural language processing for healthcare.
HIPAA compliant Alexa Skills
This blog post outlines the first HIPAA compliant skills.
This page shows Alexa resources for building Healthcare skills.
Omnichannel Conversational AI in Healthcare
Nate Treloar shares lessons learned by Orbita from years of implementing telehealth solutions with an emphasis on conversational AI